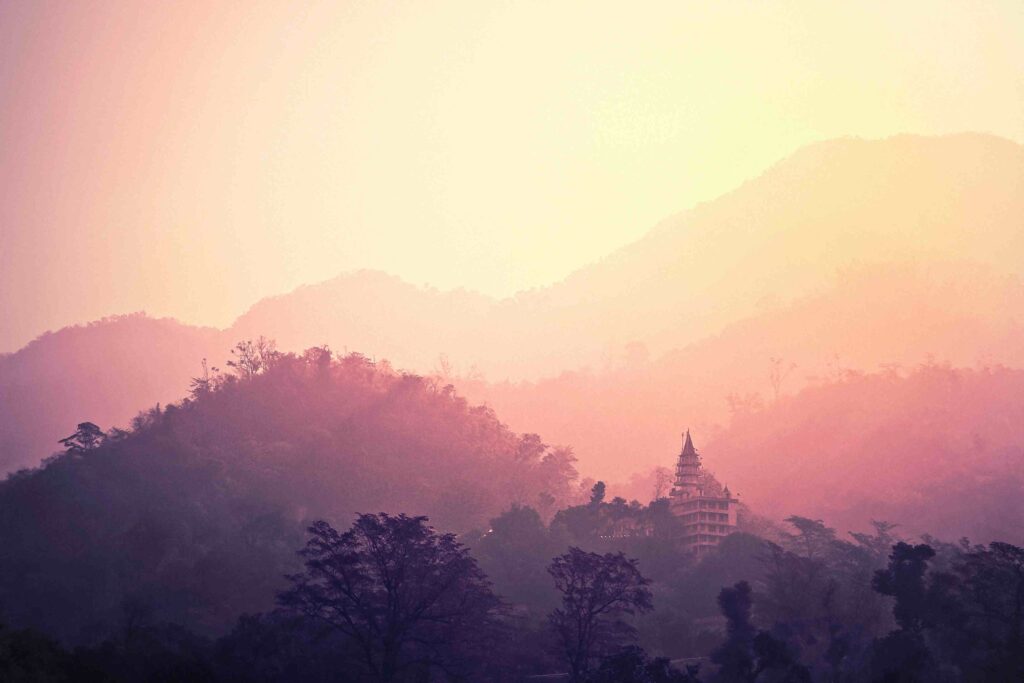
My uncle was a Forest officer in Gujarat and many a times we spent our holidays with my Aunt and Uncle in Vadodara. So, customarily in the year 1993 (I was 12 years old) as well during our summer vacations, we decided to visit my Aunt and Uncle. My Mother has 5 sisters and 1 brother which meant I have many cousins, and we were never alone going on any such trip. The trips would be anywhere between 2-4 weeks and we used to visit many places across Gujarat not limited to just Vadodara. One such place we visited was Pavagadh a hill, which is approximately 46 Km away from Vadodara. The hill has a total height of 822 meters and atop the hill there is a Maha Kali (Indian Goddess) temple, which we planned to visit.
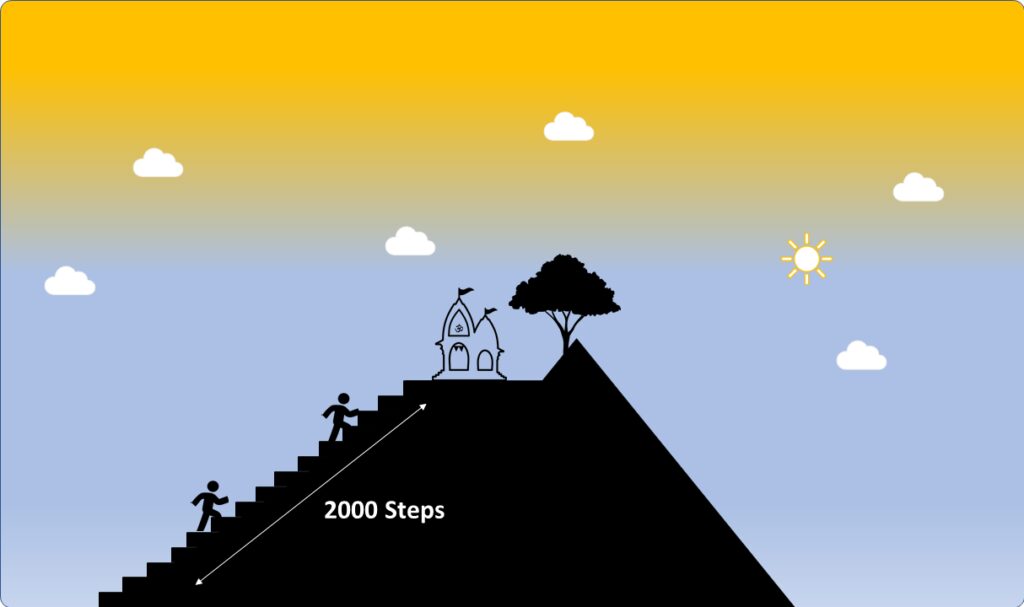
To reach the temple we had to climb approximately 2000 steps; Me, my sister, and my cousins were all in the age range of 12 – 15 years but I do not remember if were enthusiastic about doing something like this. We were pacing ourselves, counting the number of steps we walked every 10 mins. The average total time taken to climb the stairs is anywhere between 2-4 hours (120 to 240 mins). Climbing stairs is not an easy thing to do especially Two Thousand, the first one hour was possibly good as we climbed on an average 110 steps every 10 mins. We also took some breathing time in between, the next one hour was the tough as the average steps per 10 mins dropped to 75.
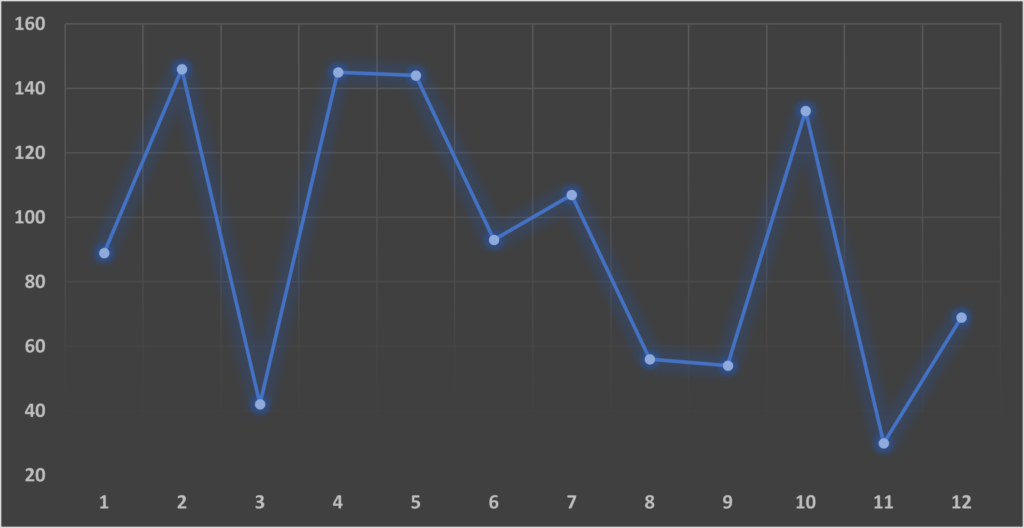
At the end of two hours, we are well completely out of breath, and we had approximately 900 steps to go. As kids we decided to challenge ourselves, we wanted to guess how many steps we would complete in the next 10 mins. We can find this out more ways than one, but at that age of twelve the simplest thing to do is just take an average of the last three values or two or four. Little did I know that this method of just taking averages has a sophisticated name “Moving Average” or MA Models. Now that I think about it could I have used some other method, let us give that a bit more thought.
Standing at the 1100th step I look back from where we started; I remember the enthusiasm at the start, the joy of reaching the 100th Step and many small things. If we had started off with say reaching 300 steps in the 1st 10 mins, then there is every chance we would have been out of breath then and there. So, what is the inference? The way we climbed the 1100 steps will have an impact on how we climb the remaining 900 steps. In terms of Machine Learning this is what we call Auto-Regression, here I can use the past values (Steps per 10 mins) to know about the future. If you notice in the Graph our pace is low in the first 10 mins then it increases in the next. Mathematically, this logic can be leveraged, and these Models are known as AR Models and are many a times also referred to Long Memory Models. Practically speaking how I took the first step will impact how I take the 100th, 200th of 500th step and all this can be represented mathematically, which is why the model is a Long Memory Model. Like in moving average, in this case as well one can consider past n values (One or two or four etc.).
As a kid, we like to experiment a lot and on similar lines, well someone decided to experiment with a combination of both the AR and MA models. If your question is, is this possible, yes, we can that is what is called an ARMA model. As a 12-year-old I studied some elements of astronomy which included Earth, Moon, the planets, the Milky way galaxy and many other points. An important aspect we studied is that the earth moves around its own axis in 24 hours and around the sun in 365 days. At any point in time the speed of the earth spinning (though we do not feel it) around the sun is same and consistent. Logically this is called Stationarity and is an important aspect in forecasting (especially time series). Mathematically we need to verify that certain parameters like mean, variance, standard deviation are constant at any point in time. Most of the times you will never have data which is stationary and one way to make the data stationary is by using specific techniques like differencing (simple example – difference of current and prior value).
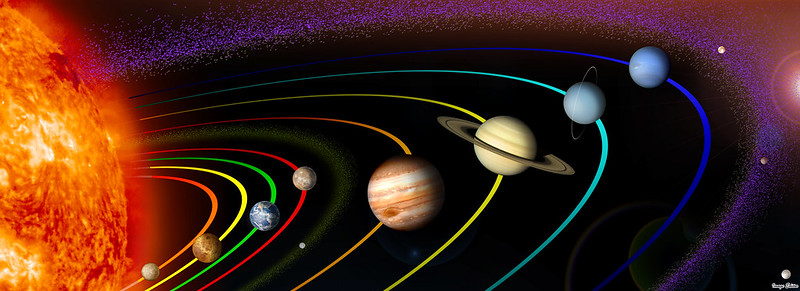
Combing all the three elements (AR + MA + Differencing) one gets a model known as ARIMA – Auto Regressive Integrated Moving Average. I went to Pavagadh during the Summer, let us say I decided to visit the temple once every month for two years. Practically speaking it might be easier to climb the stairs when the weather is cooler rather than when the sun is beating down on us. In this case we have a seasonal component as well and there is another model for this called SARIMA or Seasonal ARIMA.
I might have bored you a lot around some basic concepts around Time Series forecasting, do note while explaining the concept I have left out the mathematics to keep the article simple. Now, there are many applications of the Time Series Forecasting, assuming you have decent amount of data to work with. I have listed some of the forecasting examples in Healthcare below.
- Predict the number of patients walking into a clinic at a specific date.
- Forecast the COVID cases for the next two weeks, do note there are better models to predict epidemics other than ARIMA but people have used ARIMA.
- Forecast the number of planned surgeries for a specific month.
- Forecast the possible nurse attritions in a hospital monthly.
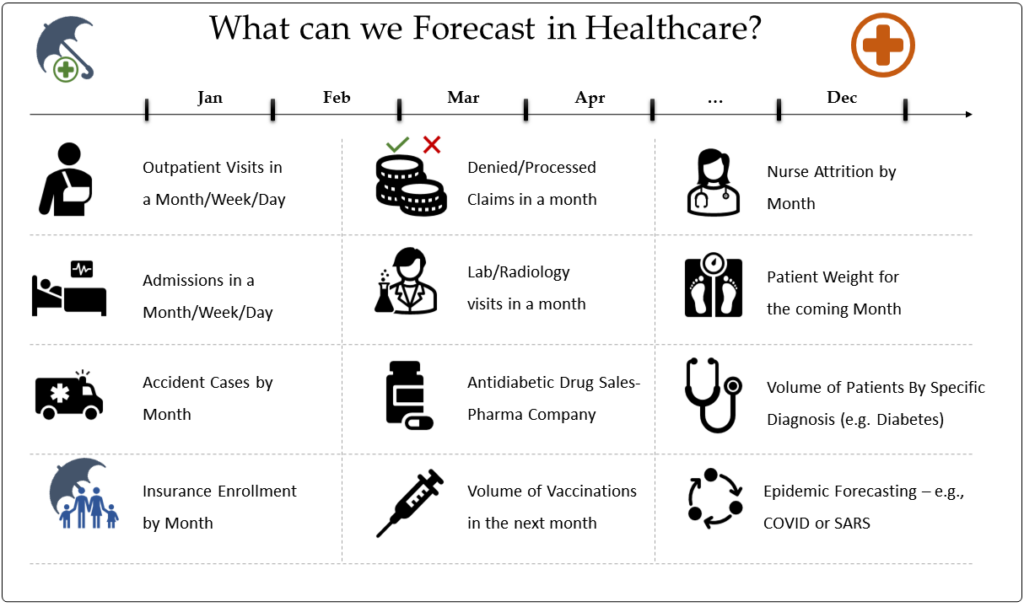
If you would like to understand Analytics in a fun and fictional way then Grab a copy of my book “Master the Mystic Arts – The Journey Begins”. If you are a Kindle Fan you can get a copy of my book on Amazon India, if you prefer the print version you can order it from Pothi. You can also explore the different elements of my book by checking this interactive Tableau Dashboard.
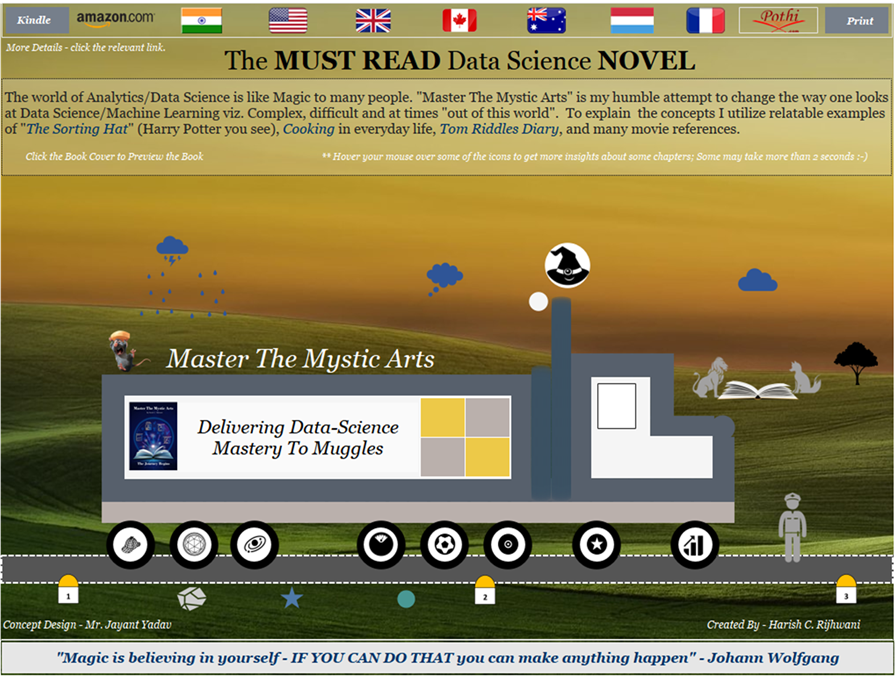
References:
- Flickr. 2021. 01 The Solar System PIA10231, mod02. [online] Available at: <https://www.flickr.com/photos/11304375@N07/2818891443> [Accessed 14 May 2021]
Super!👍
Superb explanation
Very well explained, sir👏
Opened up a new perspective to understand Data Science. Nice work. #sarima 👍
Very nicely written! It was fun reading 🙂 Keep it up!
As always putting the complex of the concept in the easiest of the way!
Very well written 👌👍